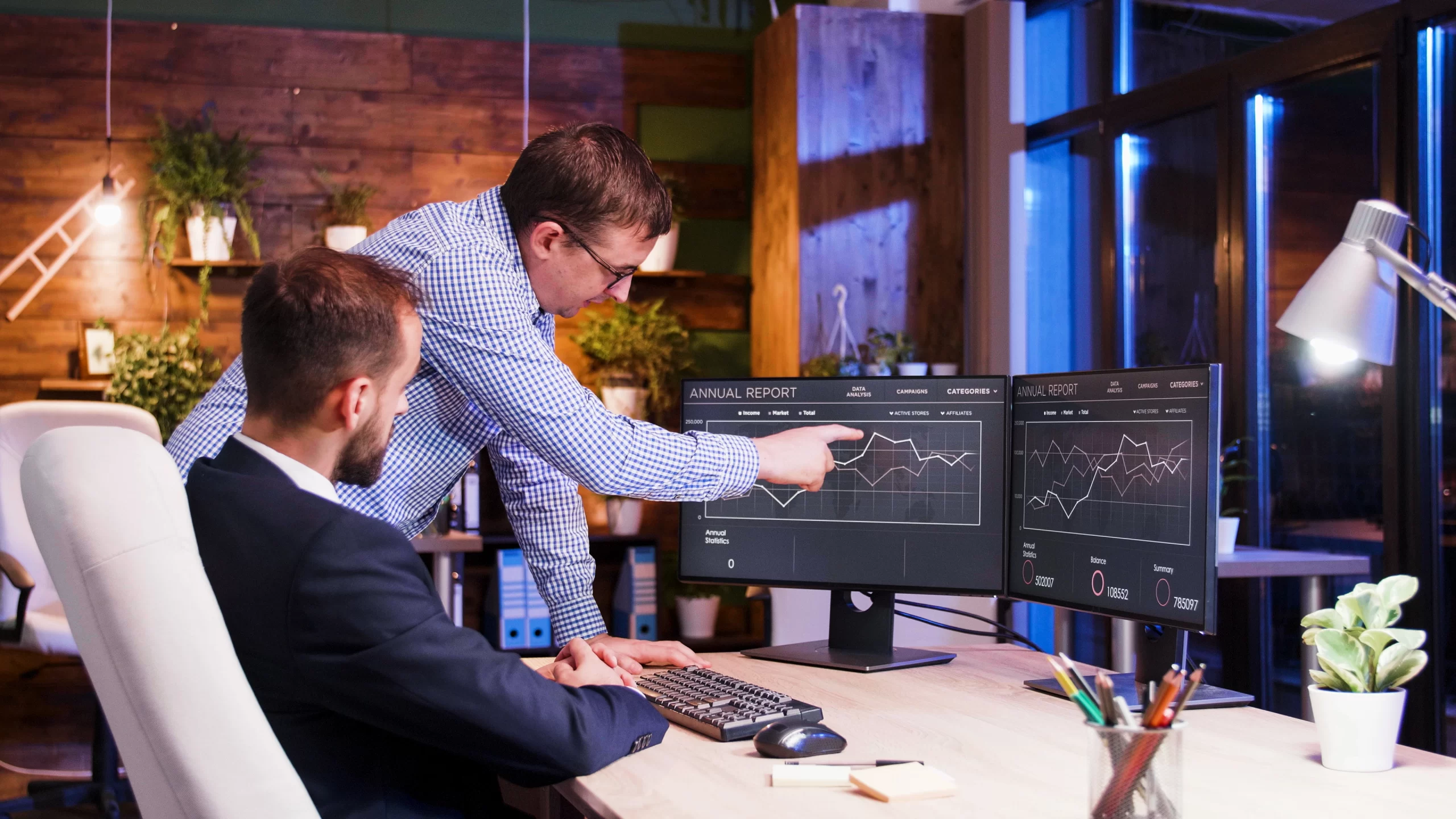
Data Science Hub
1. The Essence of Data Science
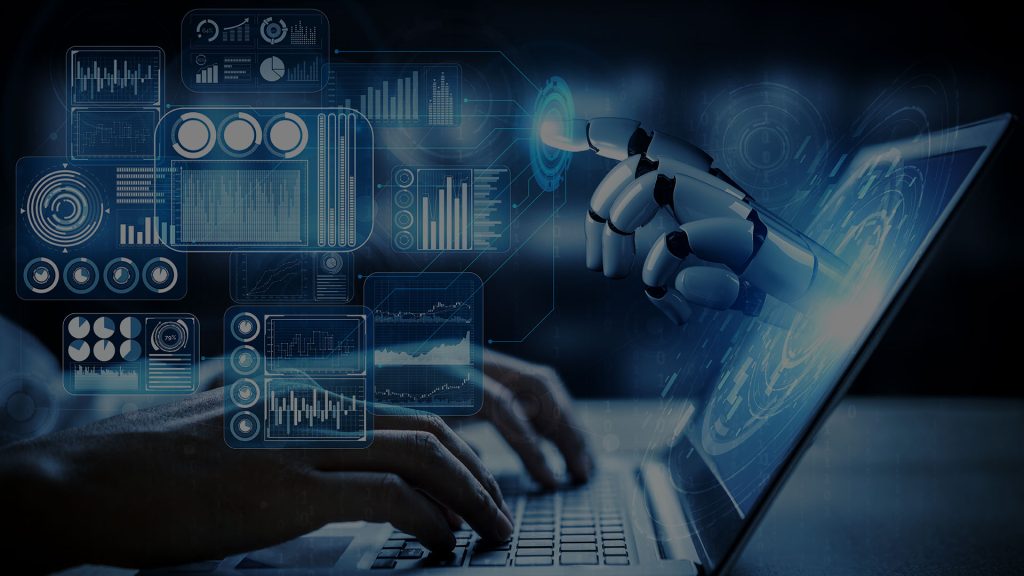
2. The Data Science Process
Data science is not just about applying complex algorithms to data; it involves a well-defined process that guides practitioners from data collection to deriving actionable insights. The process typically includes the following stages:
Data Collection: Gathering relevant data from various sources, such as databases, APIs, or sensors. Ensuring data quality and addressing issues like missing values and outliers is crucial at this stage.
Data Cleaning and Preprocessing: Raw data is often messy and inconsistent. Data scientists employ techniques to clean, transform, and structure the data, making it suitable for analysis.
Exploratory Data Analysis (EDA): This step involves visually and statistically analyzing the data to identify trends, patterns, and outliers. EDA helps in formulating hypotheses and determining the appropriate analysis methods.
Feature Engineering: Creating meaningful features or variables from the raw data to improve the performance of machine learning models. This requires domain knowledge and creative thinking.
Model Building: Developing and training machine learning models using algorithms that are tailored to the problem at hand. This step involves selecting appropriate algorithms, tuning hyperparameters, and evaluating model performance.
Model Evaluation and Validation: Testing the model’s performance on new, unseen data to ensure its generalizability. Techniques like cross-validation and metrics like accuracy, precision, and recall are used for assessment.
Insights and Interpretation: Extracting valuable insights from the model’s predictions and translating them into actionable recommendations for stakeholders.
3. Real-World Applications
The applications of data science are vast and diverse, spanning across industries:
Healthcare: Predictive analytics can help forecast disease outbreaks, while machine learning aids in diagnosing medical conditions from imaging data.
Finance: Fraud detection, credit risk assessment, and algorithmic trading are some areas benefiting from data science.
E-commerce: Personalized recommendations, demand forecasting, and customer segmentation drive the success of many e-commerce platforms.
Marketing: Customer behavior analysis, A/B testing, and sentiment analysis assist in crafting effective marketing campaigns.
Transportation: Optimization of routes, traffic prediction, and autonomous vehicles rely on data-driven insights.
4. Ethical Considerations and Challenges
While data science offers immense potential, it also raises ethical concerns regarding privacy, bias, and accountability. Biased data can lead to discriminatory algorithms, and mishandling of sensitive data can breach user privacy. Data scientists need to be vigilant in addressing these issues to ensure responsible and equitable use of data.
5. Future Trends
The field of data science continues to evolve rapidly. Advancements in deep learning, natural language processing, and reinforcement learning are pushing the boundaries of what’s possible. Moreover, the integration of data science with other technologies like the Internet of Things (IoT) and blockchain is expected to open new avenues for innovation.
Conclusion
Data science is a captivating journey that transforms raw data into insights that can drive innovation and decision-making. Its multidisciplinary nature and wide-ranging applications make it a critical tool across industries. As data continues to shape our world, mastering the art of data science becomes essential for anyone seeking to unlock the power hidden within the data-rich landscape of the 21st century. So, whether you’re a seasoned professional or just beginning to explore the world of data, remember that every data point has a story to tell—waiting to be uncovered through the lens of data science.